|
Arduino Nano BLE 33 Sense |
x 1 | |
|
MICS 4514 multi gas sensor |
x 1 | |
|
Oled monochrome display |
x 1 | |
|
Buzzer |
x 1 |
![]() |
arduino IDEArduino
|
|
![]() |
Autodesk Fusion 360Autodesk
|
Bhopal 84, detect harmful gases with machine learning and Arduino
Industries working with chemicals are always subject to leaks that could harm workers. Sometimes those leaks are formed by a specific combination of gases not suitable by off the shelf sensors but a machine learning model could be trained to identify subtle relationships between all the gas readings and calculations.
The device will read the multigas sensor x times for y seconds and then calculate min, max and average. Those values will be forwarded to the Machine Learning model for inference and then a score will be obtained for “harmful” gas 1 or “regular” gas 2.
Connections
Circuit schema will work as a reference. In detail you need to connect Buzzer to D2 and GND. Oled Screen VCC to Arduino 3.3v, GND to Arduino GND, SDA to A4, SCL to A5. Gravity Gas Sensor to Arduino 3.3v, GND, SDA to A4, SCL to A5
Data acquisition
If you are going to train a model to detect harmful gases take all the precautions like wearing a mask and ventilate the area. Several minutes are required to gather data and any person could become ill during that time.
Upload to the Arduino BLE 33 sense. Place the sensor unit close to the gas or substance and open serial monitor. You should see there multi gas sensor values as CSV records. Uncheck timestamp, then copy and paste the serial monitor screen into a text file. Save that file has harmful.csv and add this header:
timestamp CO2avg C2H5OHavg H2avg NH3avg CO2min C2H5OHmin H2min NH3min CO2max C2H5OHmax H2max NH3max
Repeat the procedure for all the gases to be detected.
Cut 20% of your data for testing and save into a different CSV file. This is useful to check later whether the machine learning model is actually doing good predictions. We know that all data came from the same gas. We train the model with some data and then we feed data not used to train the model just to make sure that those values are detected.
Model training
First check that you have all the data for training and testing uploaded with correct labels. Then go to Impulse Design, Create Impulse. What is an Impulse? An impulse takes raw data, uses signal processing to extract features, and then uses a learning block to classify new data.
In Times Series Data, we will use 1500ms Windows Size and 0,6 frecuency. Window increase is not important here, since samples are taken at exactly X seconds.
Processing block will be raw data with all axis checked. For classification we will use Keras with 2 output features: regular and harmful.
In Raw Data you can see all values for regular and harmful inside every windows size. Then you have to click Generate Features.
For NN Classifier we will use 60 training cycles, 0.0005 Learning Rate, Validation 20 and Autobalance dataset. It worked for me adding an extra layer Droput Rate 0.1 Click Start Training and check if you get good accuracy. In my case 86% good harmful detection and 96% regular gas detection.
If you are ok with results you can go to Model Testing and check the performance with new data. If there are lots of readings with wrong classification you should check again data acquisition procedure.
Using Bhopal 84
After displaying Bhopal and Edge Impulse logos, the unit will start the calibration phase. During this phase do not place any substance or gas under the sensor unit. It should read normal air conditions. As soon as this step is finished you can put the leaked substance under the sensor unit and it should be detected in 1.5 seconds. Why 1.5 seconds? 4 readings will be made to obtain mix, max and average for all gases. That information will be forwarded to the model and a classification will be returned.
The prototype is able to detect normal air conditions, a regular gas and a harmful gas.
Demo
Bhopal 84, detect harmful gases with machine learning and Arduino
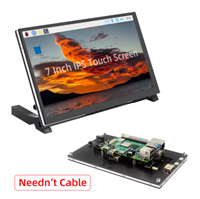
Raspberry Pi 5 7 Inch Touch Screen IPS 1024x600 HD LCD HDMI-compatible Display for RPI 4B 3B+ OPI 5 AIDA64 PC Secondary Screen(Without Speaker)
BUY NOW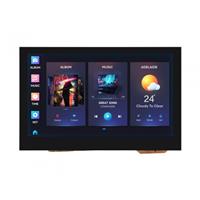
ESP32-S3 4.3inch Capacitive Touch Display Development Board, 800×480, 5-point Touch, 32-bit LX7 Dual-core Processor
BUY NOW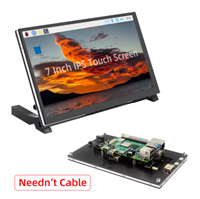
Raspberry Pi 5 7 Inch Touch Screen IPS 1024x600 HD LCD HDMI-compatible Display for RPI 4B 3B+ OPI 5 AIDA64 PC Secondary Screen(Without Speaker)
BUY NOW- Comments(0)
- Likes(0)

- 0 USER VOTES
- YOUR VOTE 0.00 0.00
- 1
- 2
- 3
- 4
- 5
- 6
- 7
- 8
- 9
- 10
- 1
- 2
- 3
- 4
- 5
- 6
- 7
- 8
- 9
- 10
- 1
- 2
- 3
- 4
- 5
- 6
- 7
- 8
- 9
- 10
- 1
- 2
- 3
- 4
- 5
- 6
- 7
- 8
- 9
- 10
More by Roni Bandini
-
Fall Detection client-server system with Machine Learning Falls could be dangerous in any situation but for certain working scenarios, consequences are defini...
-
Bhopal 84, detect harmful gases with machine learning and Arduino Industries working with chemicals are always subject to leaks that could harm workers. Sometimes tho...
-
Ibarrola, anti facial recognition servo glasses These are simple servo glasses designed to fool facial recognition software with manual and automati...
-
Bitcoin ring with Attiny85 I’ve started to think about a project that could take advantage of DigiSpark board features (reduced...
-
Dry Martini WiFi operated neon led sign English CC captions availableI like Dry Martinis. Maybe due to the cocktail glass design or to the f...
-
Vespa Diorama ESP32 NTP clock I love Italian motorcycles. I have a Ducati, I’ve rided a Guzzi to travel around Europe and I even f...
-
Swatch Retro Internet Time Clock with Xiao TFT Round Display Internet Time is a decimal time concept released in 1998 by the Swatch corporation. Instead of hours...
-
Joule Thief Components:Ferrite torroid (You can make one with an old low consumption Lamp and 2 copper wires)1k ...
-
Fall Detection client-server system with Machine Learning Falls could be dangerous in any situation but for certain working scenarios, consequences are defini...
-
Bhopal 84, detect harmful gases with machine learning and Arduino Industries working with chemicals are always subject to leaks that could harm workers. Sometimes tho...
-
Ibarrola, anti facial recognition servo glasses These are simple servo glasses designed to fool facial recognition software with manual and automati...
-
Bitcoin ring with Attiny85 I’ve started to think about a project that could take advantage of DigiSpark board features (reduced...
-
Dry Martini WiFi operated neon led sign English CC captions availableI like Dry Martinis. Maybe due to the cocktail glass design or to the f...
-
Vespa Diorama ESP32 NTP clock I love Italian motorcycles. I have a Ducati, I’ve rided a Guzzi to travel around Europe and I even f...
-
Swatch Retro Internet Time Clock with Xiao TFT Round Display Internet Time is a decimal time concept released in 1998 by the Swatch corporation. Instead of hours...
-
Joule Thief Components:Ferrite torroid (You can make one with an old low consumption Lamp and 2 copper wires)1k ...
-
Fall Detection client-server system with Machine Learning Falls could be dangerous in any situation but for certain working scenarios, consequences are defini...
-
Bhopal 84, detect harmful gases with machine learning and Arduino Industries working with chemicals are always subject to leaks that could harm workers. Sometimes tho...
-
Ibarrola, anti facial recognition servo glasses These are simple servo glasses designed to fool facial recognition software with manual and automati...
-
Bitcoin ring with Attiny85 I’ve started to think about a project that could take advantage of DigiSpark board features (reduced...
-
-
Modifying a Hotplate to a Reflow Solder Station
513 0 4 -
MPL3115A2 Barometric Pressure, Altitude, and Temperature Sensor
301 0 1 -
-
Nintendo 64DD Replacement Shell
293 0 1 -
V2 Commodore AMIGA USB-C Power Sink Delivery High Efficiency Supply Triple Output 5V ±12V OLED display ATARI compatible shark 100W
756 4 2 -
How to measure weight with Load Cell and HX711
550 0 3 -